The Importance of Labeled Image Datasets in Modern Business
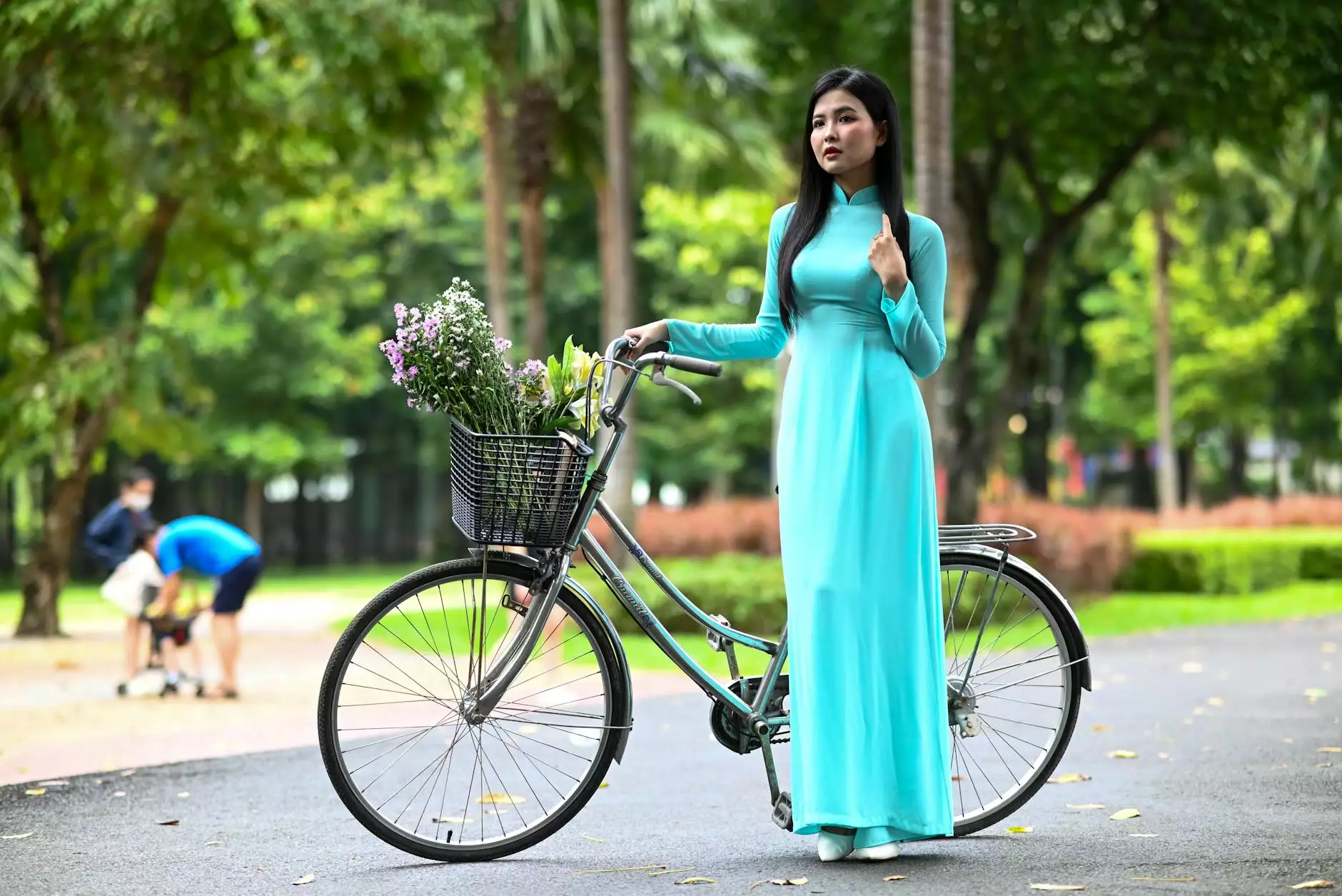
Labeled image datasets have become a cornerstone for businesses leveraging artificial intelligence (AI) and machine learning (ML) technologies. As organizations strive to enhance their operational efficiency and decision-making processes, the demand for high-quality labeled data is at an all-time high. This article delves deep into the realm of labeled image datasets, their critical roles in various industries, and how platforms like Keylabs.ai are revolutionizing the landscape of data annotation.
Understanding Labeled Image Datasets
A labeled image dataset consists of images that have been tagged with annotations describing the contents within each image. These labels can include categories, object boundaries, relationships between objects, and various attributes. The quality and accuracy of these labels are vital, as they directly influence the performance of machine learning models.
The Role of Labeled Image Datasets in Machine Learning
In machine learning, algorithms learn from data to make predictions or decisions. The training data, especially labeled datasets, plays a crucial role in how effectively these algorithms can learn. High-quality labeled image datasets serve numerous functions, including:
- Training Models: Labeled datasets are essential for training supervised machine learning models. The annotations provide the ground truth that the model uses to learn and make accurate predictions.
- Validating Accuracy: Once a model is trained, it must be validated against a set of labeled data to ensure it can accurately predict new, unseen data.
- Improving Algorithms: Continuous learning and adaptation of algorithms depend on adding diverse and well-labeled datasets to prevent overfitting and improve accuracy.
The Business Applications of Labeled Image Datasets
Labeled image datasets have a wide range of applications across multiple industries. Their versatility makes them invaluable assets for businesses aiming to harness AI capabilities. Here are some prominent sectors that greatly benefit from labeled image datasets:
1. Healthcare
In healthcare, labeled image datasets play a critical role in the development of AI-assisted diagnostic tools. For example:
- Medical imaging such as MRIs and CT scans can be annotated to train models that detect anomalies, thereby enhancing diagnostic accuracy.
- Labeled data helps in segmenting different tissues or detecting tumors, greatly assisting radiologists in their assessments.
2. Autonomous Vehicles
Autonomous driving technology relies heavily on image recognition systems for safe navigation. Key aspects include:
- Labeled datasets of road signs, pedestrians, and various driving conditions help train models to recognize and respond to the dynamic environment on roads.
- Data annotation in this field must be precise to ensure the safety and efficiency of autonomous systems.
3. Retail and E-commerce
In the retail sector, labeled image datasets enhance customer experiences and operational efficiency through:
- Visual search capabilities that allow customers to upload images and find similar products instantly.
- Inventory management systems use image recognition to monitor stocks and assist in restocking processes.
4. Agriculture
Labeled datasets are transforming how we approach farming through precision agriculture:
- Remote sensing data can be annotated to identify crop health and yield predictions, allowing farmers to make informed decisions.
- Automated systems can monitor and manage crops more effectively by recognizing patterns in labeled imagery.
Challenges in Creating High-Quality Labeled Image Datasets
While the need for labeled image datasets is clear, creating them poses several challenges:
1. Cost and Time Constraints
Data annotation can be a labor-intensive process, requiring significant investment in both time and resources.
2. Consistency and Accuracy
Maintaining a high level of consistency in labeling is crucial. Inconsistencies can lead to poor model performance and misguided insights.
3. Expertise
Sophisticated domains like medical imaging require expert annotators to ensure data accuracy, which can further inflate costs.
How Keylabs.ai is Transforming Data Annotation
Keylabs.ai provides an advanced data annotation tool and data annotation platform that addresses the challenges of creating labeled image datasets. Here’s how:
1. Automated Annotation Tools
Keylabs.ai employs AI-driven solutions to speed up the labeling process. These tools significantly reduce manual effort while ensuring precision in annotations.
2. Collaboration and Scalability
The platform allows seamless collaboration between team members, enabling companies to scale their data annotation efforts efficiently without compromising quality.
3. Quality Assurance
To combat inconsistencies, Keylabs.ai implements rigorous quality assurance protocols. Labeled data undergoes multiple validation phases to ensure it meets high accuracy standards.
Future Trends in Labeled Image Datasets
The landscape of labeled image datasets is continuously evolving. Here are some future trends to watch:
1. Increased Use of Synthetic Data
Generating synthetic datasets through simulation will become more prevalent, addressing the cost and time barriers while providing diverse training examples.
2. Crowdsourced Annotation
Leveraging crowdsourcing for data annotation can speed up the process and reduce costs, but it requires robust systems to ensure accuracy and consistency.
3. Enhanced AI Models for Annotation
As AI technology improves, expect to see more capable models that can assist in or fully automate the annotation process, thereby revolutionizing the way data is prepared for machine learning.
Conclusion
In closing, labeled image datasets are an indispensable resource for businesses across many industries. Their ability to improve AI models and drive intelligent decision-making is crucial as organizations navigate an increasingly data-driven world. Leveraging platforms like Keylabs.ai ensures businesses can effectively create and manage these datasets, providing them with a competitive edge in their respective fields. Embracing the power of labeled image datasets today will undoubtedly shape the innovations of tomorrow.