Harnessing the Power of Medical Datasets for Machine Learning in Business
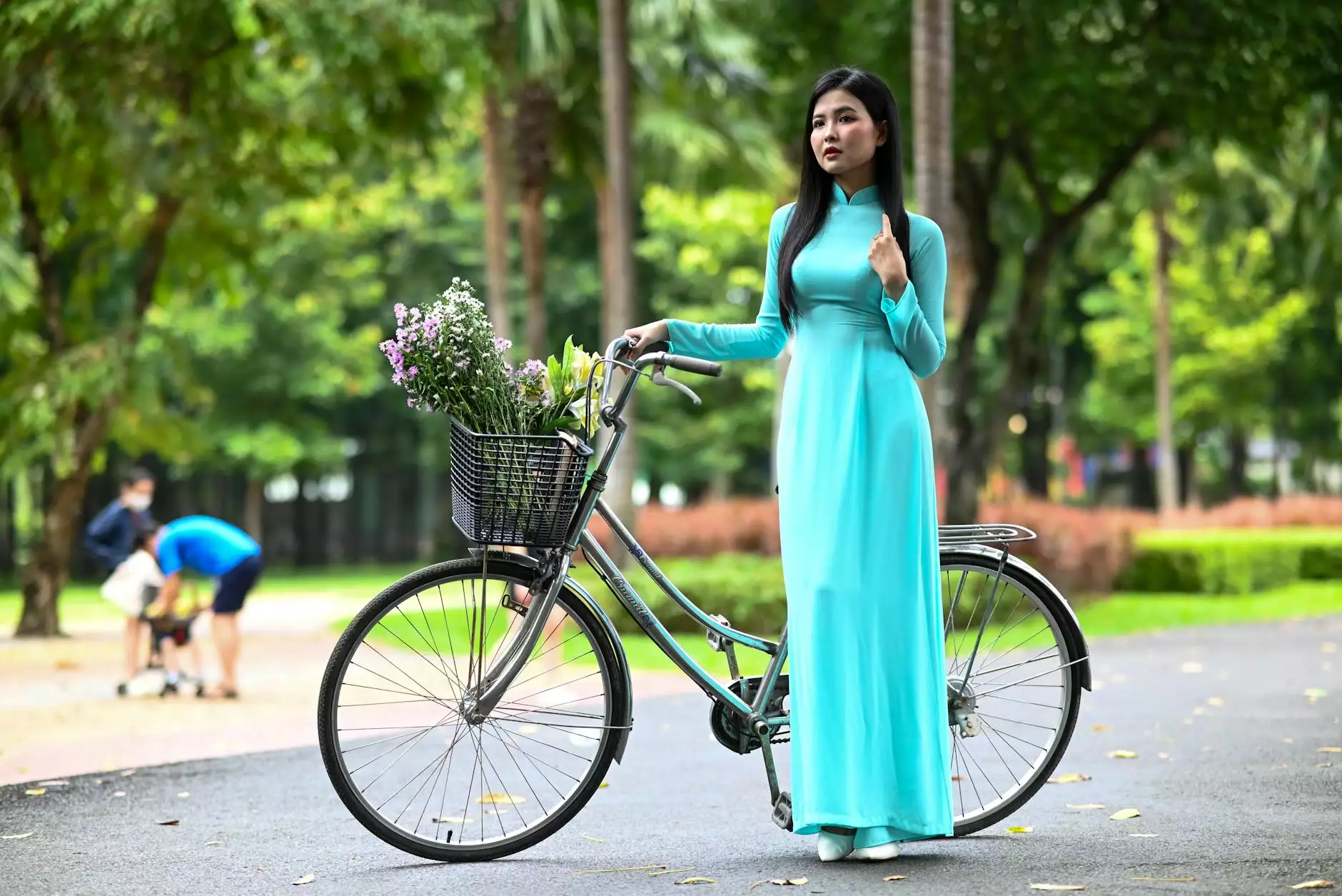
In today's digital age, the integration of technology in various sectors has led to unprecedented advancements. One of the most promising fields is the intersection of healthcare and machine learning. The use of medical datasets for machine learning is revolutionizing how businesses approach healthcare challenges, driving innovation, improving outcomes, and enhancing patient experiences.
Understanding Medical Datasets
Medical datasets are collections of data that originate from various sources within the healthcare domain. They can include patient records, clinical trial data, genomic information, health surveys, and more. The richness of this data makes it invaluable for developing machine learning models.
Types of Medical Datasets
- Electronic Health Records (EHR) - Comprehensive patient data ranging from medical history to treatment outcomes.
- Clinical Trials Data - Data collected during trials that evaluate the effects of new drugs or treatment protocols.
- Genomic Data - Information about individual genomes that can be used for personalized medicine.
- Imaging Data - Medical images (X-rays, MRIs, CT scans) used in diagnostic processes.
- Billing Data - Information related to medical billing that can provide insights into healthcare costs and patient demographics.
The Role of Machine Learning in Healthcare
Machine learning is a subset of artificial intelligence that enables systems to learn and improve from experience without explicit programming. In healthcare, machine learning algorithms analyze large volumes of data to uncover patterns, predict outcomes, and inform decision-making processes.
Applications of Machine Learning in Healthcare
- Predictive Analytics - Predicting patient outcomes and disease outbreaks based on historical data.
- Personalized Medicine - Tailoring treatment plans to individual patients based on genomic data.
- Medical Imaging Analysis - Automating the interpretation of medical images to assist radiologists.
- Drug Discovery - Streamlining the process of discovering new drugs using data-driven approaches.
- Patient Risk Stratification - Identifying high-risk patients who may require more intensive management.
Benefits of Utilizing Medical Datasets for Machine Learning
The integration of medical datasets for machine learning brings numerous advantages to businesses operating in the healthcare sector. Here are some key benefits:
Improved Decision Making
By leveraging machine learning models that are trained on rich datasets, healthcare providers can make well-informed decisions based on empirical evidence rather than subjective judgment. For instance, predictive analytics can help anticipate patient admissions, allowing hospitals to allocate resources more effectively.
Enhanced Patient Outcomes
Machine learning can facilitate tailored treatment plans that improve patient care. By analyzing patient data, healthcare professionals can deliver interventions that are specific to a patient's unique situation, thus enhancing the overall quality of care.
Cost Reduction
The automation and efficiency brought by machine learning can lead to significant cost savings. By optimizing processes such as billing, resource allocation, and patient management, healthcare businesses can lower operational costs and improve profitability.
Faster Drug Development
Utilizing machine learning algorithms on clinical trial datasets can significantly accelerate the drug discovery process. Companies can identify potential drug candidates more quickly and efficiently, reducing the time to market for new therapies.
Challenges in Utilizing Medical Datasets
While the benefits are clear, there are several challenges that businesses must navigate when working with medical datasets for machine learning:
Data Privacy and Security
Given the sensitive nature of health information, data privacy regulations (like HIPAA in the United States) pose a significant challenge. Businesses must ensure that they comply with these regulations while leveraging data for analysis and insights.
Data Quality and Standardization
Medical datasets often come from diverse sources, leading to discrepancies in data quality and format. Ensuring that data is standardized and of high quality is crucial for the success of machine learning initiatives.
Interoperability Issues
Healthcare systems often use different technologies and platforms that may not easily connect with one another. This lack of interoperability can hinder the effective use of datasets across systems.